PI Probaligence Gmbh
PI Probaligence Gmbh
THE RESOURCE-EFFICIENT ADVANTAGES OF THE PROBABILISTIC MACHINE LEARNING SOFTWARE STOCHOS© FOR DEDICATED SIMULATION APPLICATIONS
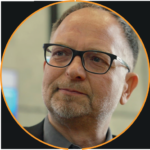
„If research succeeds in expanding machine learning methods to include probabilistic analysis, then artifi cial intelligence will be-come more and more similar to human intelligence and therefore more effi cient and more reliable in forecasting.“
Prof. Dr.-Ing. Dirk Roos, Co-Founder PI Probaligence
In the field of automotive engineering, advanced simulation technologies are crucial for improving vehicle design, performance, and safety. The choice of machine learning algorithms for these simulations affects their efficiency and accuracy. While deep neural networks (DNNs) are powerful in handling big data, our resource-efficient probabilistic machi-ne learning algorithm off er several advantages for specific simulation tasks, as for example it provides a measure of uncertainty in its predictions. This is particularly useful in simulation applications where knowing the confidence and variability of results is important. For example, in crash simulations or fatigue analysis, understanding uncertainty helps engineers make better decisions and create designs that consider various risks and real-world variations.
While large DNNs need a lot of computational re-sources for training because of their deep network structure, probabilistic solutions can achieve the same results with a fraction on computational re-sources.
Another advantage is the ability to work well with smaller datasets. In many engineering situations, gathering substantial amounts of training data can be di ffi cult or expensive. Probabilistic models can use the available data more efficiently, providing strong predictions without needing large datasets, which is often a problem for DNNs.
PI’S INNOVATIVE APPROACH WITH STOCHOS© ML SOFTWARE
PI Probaligence GmbH, an innovative company in probabilistic AI solutions and partner in the CADFEM Group, has developed an advanced set of machi-ne learning tools called STOCHOS©, which uses a probabilistic machine learning algorithm approach. STOCHOS© performs sample- and resource-efficient, expert-independent probabilistic machine learning, using both existing data and planning new data collection. STOCHOS© can learn from all kinds of data shapes and formats, e.g. transient 2D or 3D FEM / CFD and re-place expensive simulations like, for example, aerodynamic simulations. Even signal data like in NVH tasks are possible. It does not matter whether the data comes from real experiments or simulations, showing its high flexibility.
STOCHOS© supports all three kinds of machine learning, supervised, unsupervised and reinforcement learning. In supervised learning, it is excellent for replacing simulation solvers and performing optimization. Un-supervised learning would be for example predictive maintenance tasks where the model analyzes anomalies in sensor data. Reinforcement learning tasks cover all kinds of real time control tasks like in robotics or for example autonomous driving. The software works on various hardware platforms, including CPU, GPU, and microcontrollers, allowing for real-time responses.
A key feature of STOCHOS© is its multi-fidelity computation capability, which increases computational efficiency or handles very complex problems with limited computational resources. It allows to combine different fidelity levels of data sources like for example coarse and fi ne mesh simulation results or to combine experimental and simulation data within one model to solve simulation calibration tasks. This makes STOCHOS© exceptionally good at managing many different simulations needs without losing performance or accuracy.
PI Probaligence’s STOCHOS© software combines the best features of Deep Learning (DL) and Gaussian Processes (GP) while avoiding their downsides. Unlike large ML companies that often rely on deep learning and need extensive hyperparameter tuning to fi nd the best network setup, STOCHOS© does not need any kind of hyperparameter training. In addition, the data stays securely within the customer’s premises, avoiding the need for expensive and resource-heavy cloud computing solutions.
ADDRESSING KEY ISSUES IN CURRENT ML PRACTICES
I Probaligence’s approach addresses several key prob-lems with traditional deep learning models and cloud-ba-sed solutions:
Data Privacy: Data does not leave the customer’s company, ensuring higher privacy and security.
Data Ownership: External data center providers do not learn from customers‘ data, keeping proprietary information safe.
Cost Efficiency: No need for high-performance computing (HPC) reduces overall costs.
Environmental Impact: Lower computational needs mean less electric power use, lower CO2 emissions, and less cooling water usage.
STOCHOS© is already trusted and used by major auto-motive OEMs and tier-1 suppliers, proving its eff ective-ness and reliability in real-world applications.
In conclusion, company PI Probaligence’s resource-ef-fi cient probabilistic machine learning algorithm off ers many advantages over deep neural networks for simu-lation tasks in automotive engineering. With our innova-tion, the engineering industry can achieve more e ffi cient, accurate, and sustainable simulations, helping to advan-ce automotive technology.
Adresse
-
Innovationspark Augsburg
Am Technologiezentrum 4
86159 Augsburg, Germany - +46 160 45 090 24
-
info@probaligence.com
help@probaligence.com
Social Networks
- yourfbusername
- @twitterhandle
- insta_account
- plusprofilename
- username
Link Liste
PI Probaligence GmbH
(c) 2023 All Rights Reserved